CHI Preview: HCII Researchers Develop Novel Tool for Modeling Human Behavior
News
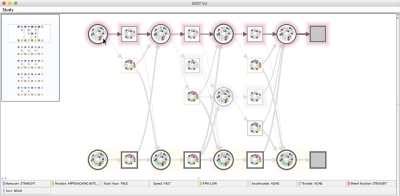
Everyone has some kind of routine. Whether it's how you get ready each morning or how you drive your car, routines are behavior blueprints that help us purposefully accomplish repetitive tasks. Modeling those routines, though, is anything but mundane. It provides important insight into situations where those routines occur, and may help people change their habits or improve their efficiency.
One problem with current modeling algorithms is that they don't explain the causal relationship between situations and actions — the events or occurrences in someone's life that trigger a routine activity. Understanding this information, along with variations that occur in routine behavior, would allow researchers to develop new technologies to improve peoples' lives, like helping them drive more safely or coordinate complex commuting schedules.
Researchers from Carnegie Mellon University's Human-Computer Interaction Institute (HCII) have developed a new method for automatically extracting and modeling routines that solves this problem. In their paper, "Modeling and Understanding Human Routine Behavior," Ph.D. student Nikola Banovic, alumnus Tofi Buzali, Professor Jennifer Mankoff, Department Head Anind Dey, and the French National Institute for Computer Science and Applied Mathematics' Fanny Chevalier outline this method. They'll present their work, which won an honorable mention award, at the upcoming Association for Computing Machinery Conference on Human Factors in Computing Systems (CHI 2016), May 7–12 in San Jose, Calif.
Using two datasets — one that included information about peoples' daily schedules and commutes, and another that described driving habits — the research team showed that the byproducts of an existing decision-theoretic algorithm used to predict peoples' activity actually encoded the causal relationship between routine actions and the context in which people perform those actions.
"As researchers, we are able to collect large amounts of behavior data, but up until now we have used this data in a very limited way to classify or predict human behavior," Banovic said. "Our modeling approach is a step toward novel quantitative methods that not only expand our ability to understand routine behaviors, but also to enable technology that can reason about and act on human routines in a principled way."
The work also has implications for the future. For example, future smart agents can use this modeling approach to detect aggressive driving behavior and provide the driver with feedback. "Such technologies can have a positive effect on society by making people healthier, safer and more efficient in their routine tasks," they said.
You can read the full paper to learn more about this research project. To see other research the HCII will present during CHI 2016, read our CHI preview on healthcare decision support tools or our story outlining the HCII's seven Best of CHI award papers.
Story by Susie Cribbs (DC 2000, 2006)