HCII Research Accepted to CHI 2020
News
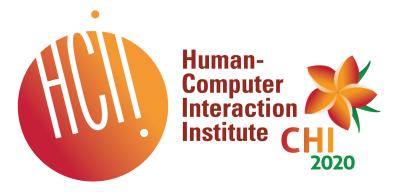
The international ACM CHI Conference on Human Factors in Computing Systems (also known as CHI) was scheduled to take place this week in Honolulu, Hawaii.
CHI 2020 was expecting its second to largest crowd ever, but the premier international conference on Human-Computer Interaction was canceled due to the coronavirus pandemic.
"CMU HCII has continued to do amazing things in the face of sheltering in place," said HCII Director, Jodi Forlizzi. "Over the past month, our community has continued to educate, research, publish, interact, and smile, even at a distance. Although we cannot celebrate CHI together in Hawaii this year, I am proud, as always, of the HCII's achievements."
Although there won't be a physical or virtual conference to attend this week, we will still highlight the awards, papers and videos from Carnegie Mellon University that were accepted to CHI 2020, including:
CHI Academy Award
Professor Jason Hong has been named to the 2020 CHI Academy, an honorary group of individuals who have made substantial contributions to the field of human-computer interaction (HCI). The distribution of these 2020 CHI Academy awards has been postponed, but will be presented at a later date.
Virtual Talks and Blog Posts
Although there is no official virtual CHI conference, the HCII is sharing prerecorded talks and blog posts of the work our authors would have presented in Hawaii this week.
Accepted Papers
Carnegie Mellon University authors contributed to more than 40 accepted papers this year, the most of any organization. Human-Computer Interaction Institute researchers were authors on four Best Paper awards and six Honorable Mention awards.
A full list of papers with CMU contributing authors is available below.
Best Paper Awards (4)
- "Critical Race Theory for HCI" -- Best Paper Award
-
[Ihudiya Finda Ogbonnaya-Ogburu, Angela D.R. Smith, Alexandra To]* & Kentaro Toyama
Abstract:
The human-computer interaction community has made some efforts toward racial diversity, but the outcomes remain meager. We introduce critical race theory and adapt it for HCI to lay a theoretical basis for race-conscious efforts, both in research and within our community. Building on the theory's original tenets, we argue that racism is pervasive in everyday socio-technical systems; that the HCI community is prone to "interest convergence", where concessions to inclusion require benefits to those in power; and that the neoliberal underpinnings of the technology industry itself propagate racism. Critical race theory uses storytelling as a means to upend deep-seated assumptions, and we relate several personal stories to highlight ongoing problems of race in HCI. The implications: all HCI research must be attuned to issues of race; participation of underrepresented minorities must be sought in all of our activities; and as a community, we cannot become comfortable while racial disparities exist. - "Co-Designing Checklists to Understand Organizational Challenges and Opportunities around Fairness in AI" -- Best Paper Award
-
Michael A. Madaio, Luke Stark, Jennifer Wortman Vaughan & Hanna Wallach
Abstract:
"Many organizations have published principles intended to guide the ethical development and deployment of AI systems; however, their abstract nature makes them difficult to operationalize. Some organizations have therefore produced AI ethics checklists, as well as checklists for more specific concepts, such as fairness, as applied to AI systems. But unless checklists are grounded in practitioners' needs, they may be misused. To understand the role of checklists in AI ethics, we conducted an iterative co-design process with 48 practitioners, focusing on fairness. We co-designed an AI fairness checklist and identified desiderata and concerns for AI fairness checklists in general. We found that AI fairness checklists could provide organizational infrastructure for formalizing ad-hoc processes and empowering individual advocates. We highlight aspects of organizational culture that may impact the efficacy of AI fairness checklists, and suggest future design directions.
- "Wireality: Enabling Complex Tangible Geometries in Virtual Reality with Worn Multi-String Haptics" -- Best Paper Award
-
Cathy Fang, Yang Zhang, Matthew Dworman & Chris Harrison
Abstract:
Today's virtual reality (VR) systems allow users to explore immersive new worlds and experiences through sight. Unfortunately, most VR systems lack haptic feedback, and even high-end consumer systems use only basic vibration motors. This clearly precludes realistic physical interactions with virtual objects. Larger obstacles, such as walls, railings, and furniture are not simulated at all. In response, we developed Wireality, a self-contained worn system that allows for individual joints on the hands to be accurately arrested in 3D space through the use of retractable wires that can be programmatically locked. This allows for convincing tangible interactions with complex geometries, such as wrapping fingers around a railing. Our approach is lightweight, low-cost, and low-power, criteria important for future, worn consumer uses. In our studies, we further show that our system is fast-acting, spatially-accurate, high-strength, comfortable, and immersive.Video:
- "Beyond the Prototype: Understanding the Challenge of Scaling Hardware Device Production" -- Best Paper Award
-
Rushil Khurana & Steve Hodges
Abstract:
The hardware research and development communities have invested heavily in tools and materials that facilitate the design and prototyping of electronic devices. Numerous easy-to-access and easy-to-use tools have streamlined the prototyping of interactive and embedded devices for experts and led to a remarkable growth in non-expert builders. However, there has been little exploration of challenges associated with moving beyond a prototype and creating hundreds or thousands of exact replicas – a process that is still challenging for many. We interviewed 25 individuals with experience taking prototype hardware devices into low volume production. We systematically investigated the common issues faced and mitigation strategies adopted. We present our findings in four main categories: (1) gaps in technical knowledge; (2) gaps in non-technical knowledge; (3) minimum viable rigor in manufacturing preparation; and (4) building relationships and a professional network. Our study unearthed several opportunities for new tools and processes to support the transition beyond a working prototype to cost effective low-volume manufacturing. These would complement the aforementioned tools and materials that support design and prototyping.Video:
Honorable Mention Awards (6)
-
"How Domain Experts Create Conceptual Diagrams and Implications for Tool Design" -- Honorable Mention Award
-
Dor Ma’ayan, Wode Ni, Katherine Ye, Chinmay Kulkarni & Joshua Sunshine
Abstract:
Conceptual diagrams are used extensively to understand abstract relationships, explain complex ideas, and solve difficult problems. To illustrate concepts effectively, experts find appropriate visual representations and translate concepts into concrete shapes. This translation step is not supported explicitly by current diagramming tools. This paper investigates how domain experts create conceptual diagrams via semi-structured interviews with 18 participants from diverse backgrounds. Our participants create, adapt, and reuse visual representations using both sketches and digital tools. However, they had trouble using current diagramming tools to transition from sketches and reuse components from earlier diagrams. Our participants also expressed frustration with the slow feedback cycles and barriers to automation of their tools. Based on these results, we suggest four opportunities of diagramming tools exploration support, representation salience, live engagement, and vocabulary correspondence that together enable a natural diagramming experience. Finally, we discuss possibilities to leverage recent research advances to develop natural diagramming tools.
- "Re-examining Whether, Why, and How Human-AI Interaction Is Uniquely Difficult to Design" -- Honorable Mention Award
-
Qian Yang, Aaron Steinfeld, Carolyn Rosé & John Zimmerman
Abstract:
Artificial Intelligence (AI) plays an increasingly important role in improving HCI and user experience. Yet many challenges persist in designing and innovating valuable human-AI interactions. For example, AI systems can make unpredictable errors, and these errors damage UX and even lead to undesired societal impact. However, HCI routinely grapples with complex technologies and mitigates their unintended consequences. What makes AI different? What makes human-AI interaction appear particularly difficult to design? This paper investigates these questions. We synthesize prior research, our own design and research experience, and our observations when teaching human-AI interaction. We identify two sources of AI's distinctive design challenges: 1) uncertainty surrounding AI's capabilities, 2) AI's output complexity, spanning from simple to adaptive complex. We identify four levels of AI systems. On each level, designers encounter a different subset of the design challenges. We demonstrate how these findings reveal new insights for designers, researchers, and design tool makers in productively addressing the challenges of human-AI interaction going forward.
- "Conversational Agency in Augmentative and Alternative Communication" -- Honorable Mention Award
-
Stephanie Valencia, Amy Pavel, Jared Santa Maria, Seunga (Gloria) Yu, Jeffrey P. Bigham & Henny Admoni
Abstract:
Augmented communicators (ACs) use augmentative and alternative communication (AAC) technologies to speak. Prior work in AAC research has looked to improve efficiency and expressivity of AAC via device improvements and user training. However, ACs also face constraints in communication beyond their device and individual abilities such as when they can speak, what they can say, and who they can address. In this work, we recast and broaden this prior work using conversational agency as a new frame to study AC communication. We investigate AC conversational agency with a study examining different conversational tasks between four triads of expert ACs, their close conversation partners (paid aide or parent), and a third party (experimenter). We define metrics to analyze AAC conversational agency quantitatively and qualitatively. We conclude with implications for future research to enable ACs to easily exercise conversational agency.
- "Twitter A11y: Making Images on Social Media Accessible" -- Honorable Mention Award
-
Cole Gleason, Amy Pavel, Emma McCamey, Christina Low, Patrick Carrington, Kris M Kitani & Jeffrey P Bigham
Abstract:
Social media platforms are integral to public and private discourse, but are becoming less accessible to people with vision impairments due to an increase in user-posted images. Some platforms (i.e. Twitter) let users add image descriptions (alternative text), but only 0.1% of images include these. To address this accessibility barrier, we created Twitter A11y, a browser extension to add alternative text on Twitter using six methods. For example, screenshots of text are common, so we detect textual images, and create alternative text using optical character recognition. Twitter A11y also leverages services to automatically generate alternative text or reuse them from across the web. We compare the coverage and quality of Twitter A11y's six alt-text strategies by evaluating the timelines of 50 self-identified blind Twitter users. We find that Twitter A11y increases alt-text coverage from 7.6% to 78.5%, before crowdsourcing descriptions for the remaining images. We estimate that 57.5% of returned descriptions are high-quality. We then report on the experiences of 10 participants with visual impairments using the tool during a week-long deployment. Twitter A11y increases access to social media platforms for people with visual impairments by providing high-quality automatic descriptions for user-posted images.
- "Keeping Community in the Loop: Understanding Wikipedia Stakeholder Values for Machine Learning-Based Systems" -- Honorable Mention Award
-
C. Estelle Smith, Bowen Yu, Anjali Srivastava, Aaron Halfaker, Loren Terveen & Haiyi Zhu
Abstract:
On Wikipedia, sophisticated algorithmic tools are used to assess the quality of edits and take corrective actions. However, algorithms can fail to solve the problems they were designed for if they conflict with the values of communities who use them. In this study, we take a Value-Sensitive Algorithm Design approach to understanding a community-created and -maintained machine learning-based algorithm called the Objective Revision Evaluation System (ORES)---a quality prediction system used in numerous Wikipedia applications and contexts. Five major values converged across stakeholder groups that ORES (and its dependent applications) should: (1) reduce the effort of community maintenance, (2) maintain human judgement as the final authority, (3) support differing peoples' differing workflows, (4) encourage positive engagement with diverse editor groups, and (5) establish trustworthiness of people and algorithms within the community. We reveal tensions between these values and discuss implications for future research to improve algorithms like ORES.
- "Automated Class Discovery and One-Shot Interactions for Acoustic Activity Recognition" -- Honorable Mention Award
-
Jason Wu, Chris Harrison, Jeffrey P. Bigham & Gierad Laput
Abstract:
Acoustic activity recognition has emerged as a foundational element for imbuing devices with context-driven capabilities, enabling richer, more assistive, and more accommodating computational experiences. Traditional approaches rely either on custom models trained in situ, or general models pre-trained on preexisting data, with each approach having accuracy and user burden implications. We present Listen Learner, a technique for activity recognition that gradually learns events specific to a deployed environment while minimizing user burden. Specifically, we built an end-to-end system for self-supervised learning of events labelled through one-shot interaction. We describe and quantify system performance 1) on preexisting audio datasets, 2) on real-world datasets we collected, and 3) through user studies which uncovered system behaviors suitable for this new type of interaction. Our results show that our system can accurately and automatically learn acoustic events across environments (e.g., 97% precision, 87% recall), while adhering to users' preferences for non-intrusive interactive behavior.
Video:
- "An Interaction Design for Machine Teaching to Develop AI Tutors"
-
Daniel Weitekamp, Erik Harpstead, Ken R. Koedinger
Abstract:
Intelligent tutoring systems (ITSs) have consistently been shown to improve the educational outcomes of students when used alone or combined with traditional instruction. However, building an ITS is a time-consuming process which requires specialized knowledge of existing tools. Extant authoring methods, including the Cognitive Tutor Authoring Tools' (CTAT) example-tracing method and SimStudent's Authoring by Tutoring, use programming-by-demonstration to allow authors to build ITSs more quickly than they could by hand programming with model-tracing. Yet these methods still suffer from long authoring times or difficulty creating complete models. In this study, we demonstrate that Simulated Learners built with the Apprentice Learner (AL) Framework can be combined with a novel interaction design that emphasizes model transparency, input flexibility, and problem solving control to enable authors to achieve greater model completeness in less time than existing authoring methods. - "Bot in the Bunch: Facilitating Discussion in Group Chat by Improving Efficiency and Participation with a Chatbot"
-
Soomin Kim, Jinsu Eun, Changhoon Oh, Bongwon Suh, Joonwan Lee
Abstract:
Although group chat discussions are prevalent in daily life, they have a number of limitations. When discussing in a group chat, reaching a consensus often takes time, members contribute unevenly to the discussion, and messages are unorganized. Hence, we aimed to explore the feasibility of a facilitator chatbot agent to improve group chat discussions. We conducted a needfinding survey to identify key features for a facilitator chatbot. We then implemented GroupfeedBot, a chatbot agent that could facilitate group discussions by managing the discussion time, encouraging members to participate evenly, and organizing members' opinions. To evaluate GroupfeedBot, we performed preliminary user studies that varied for diverse tasks and different group sizes. We found that the group with GroupfeedBot appeared to exhibit more diversity in opinions even though there were no differences in output quality and message quantity. On the other hand, GroupfeedBot promoted members' even participation and effective communication for the medium-sized group. - "Celebrating Everyday Success: Improving Engagement and Motivation using a System for Recording Daily Highlights"
-
Daniel Avrahami, Kristin Williams, Matthew L. Lee, Nami Tokunagga, Yulius Tjahjadi, Jennifer Marlow
Abstract:
The demands of daily work offer few opportunities for workers to take stock of their own progress, big or small, which can lead to lower motivation, engagement, and higher risk of burnout. We present Highlight Matome, a personal online tool that encourages workers to quickly record and rank a single work highlight each day, helping them gain awareness of their own successes. We describe results from a field experiment investigating our tool's effectiveness for improving workers' engagement, perceptions, and affect. Thirty-three knowledge workers in Japan and the U.S. used Highlight Matome for six weeks. Our results show that using our tool for less than one minute each day significantly increased measures of work engagement, dedication, and positivity. A qualitative analysis of the highlights offers a window into participants' emotions and perceptions. We discuss implications for theories of inner work life and worker well-being. - "Choice of Voices: A Large-Scale Evaluation of Text-to-Speech Voice Quality for Long-Form Content"
-
Julia Cambre, Jessica Colnago, Jim Maddock, Janice Tsai, Jofish Kaye
Abstract:
The advancement of text-to-speech (TTS) voices and a rise of commercial TTS platforms allow people to easily experience TTS voices across a variety of technologies, applications, and form factors. As such, we evaluated TTS voices for long-form content: not individual words or sentences, but voices that are pleasant to listen to for several minutes at a time. We introduce a method using a crowdsourcing platform and an online survey to evaluate voices based on listening experience, perception of clarity and quality, and comprehension. We evaluated 18 TTS voices, three human voices, and a text-only control condition. We found that TTS voices are close to rivaling human voices, yet no single voice outperforms the others across all evaluation dimensions. We conclude with considerations for selecting text-to-speech voices for long-form content.Project Webpage: https://ttschoice.github.io/
- "Collective Support and Independent Learning with Voice-Based Literacy Technology in Rural Communities"
-
Michael A. Madaio, Evelyn Yarzebinski, Vikram Kamath, Benjamin D. Zinszer, Joelle Hannon-Cropp, Fabrice Tanoh, Yapo Hermann Akpe, Axel Blahoua Seri, Kaja K. Jasińska, Amy Ogan
Abstract:
Access to literacy is critical to children's futures, but formal education may be insufficient for fostering early literacy, especially in low-resource contexts. Educational technologies used at home may be able to help, but it is unclear whether or how children (and families) will use such technologies at home in rural communities, particularly in low-literate families. In this paper, we investigate these questions with a voice-based literacy technology deployed with families in 8 rural communities in Côte d'Ivoire for 4 months. We use interviews and observations with 37 families to investigate motivations, methods, and barriers for rural families' engagement with a literacy technology accessible via feature phones. We contribute insights into how families view digital literacy as a learning goal, leverage networks of supporters, and over time, transition from explicit to implicit support for children's learning. - "Designing Games for Healthy Sleep"
-
Juliet Pesateri, Judith Leng, Qian Wang, Xiangzhu Chen, Jessica Hammer
Abstract:
A sleep deficit has far-reaching consequences, but for many people, healthy sleep is not a priority or a possibility. We explore the potential for "sleepy games" as a genre of transformational games. To explore this design space, we prototyped nine games through an iterative design process. Based on analysis of design decisions and the games as artifacts, we identify seven design challenges for sleepy games: agency and control; physiological and mental arousal; intervention timing; social embeddedness; multisensory experience; vulnerability; and identity and values. We expand on three games with playtesting to show how these design challenges unfold for players in practice, show the impact on players' lives, and discuss sleepy games as creative, social, and situated practices. - "Digital Fabrication Tools at Work: Probing Professionals' Current Needs and Desired Futures"
-
Nur Yildirim, James McCann, John Zimmerman
Abstract:
Digital fabrication tools have transformed how people work in micro- and small-scale manufacturing settings. While increasing efficiency and precision, these tools raise concerns around user agency and control. This paper describes an exploratory study investigating the felt work experience and desired futures of professionals who use fabrication tools. We conducted co-design workshops with 23 professionals who use 3D printers, laser cutters, and CNC routers. We probed about current practices; machine awareness and autonomy; and user agency. Our findings reveal that current tools are not very professional. They are unreliable and untrustworthy. Participants desired smarter tools that can actively prevent errors and perform self-calibration and self-maintenance. They had few concerns that more intelligence would impact agency. They desired tools that could negotiate trade-offs between time, cost, and quality; and that can operate as super-human shop assistants. We discuss the implications of these findings as opportunities for research that can improve professionals' work experience. - "Digital Ventriloquism: Giving Voice to Everyday Objects"
-
Yasha Iravantchi, Mayank Goel, Chris Harrison
Abstract:
Smart speakers with voice agents are becoming increasingly common. However, the agent's voice always emanates from the device, even when that information is contextually and spatially relevant elsewhere. Digital Ventriloquism allows smart speakers to render sound onto everyday objects, such that it appears they are speaking and are interactive. This can be achieved without any modification of objects or the environment. For this, we used a highly directional pan-tilt ultrasonic array. By modulating a 40 kHz ultrasonic signal, we can emit sound that is inaudible "in flight" and demodulates to audible frequencies when impacting a surface through acoustic parametric interaction. This makes it appear as though the sound originates from an object and not the speaker. We ran a study in which we projected speech onto five objects in three environments, and found that participants were able to correctly identify the source object 92% of the time and correctly repeat the spoken message 100% of the time, demonstrating our digital ventriloquy is both directional and intelligible. - "Disseminating Research News in HCI: Perceived Hazards, How-To's, and Opportunities for Innovation"
-
C. Estelle Smith, Eduardo Nevarez, Haiyi Zhu
Abstract:
Mass media afford researchers critical opportunities to disseminate research findings and trends to the general public. Yet researchers also perceive that their work can be miscommunicated in mass media, thus generating unintended understandings of HCI research by the general public. We conduct a Grounded Theory analysis of interviews with 12 HCI researchers and find that miscommunication can occur at four origins along the socio-technical infrastructure known as the Media Production Pipeline (MPP) for science news. Results yield researchers' perceived hazards of disseminating their work through mass media, as well as strategies for fostering effective communication of research. We conclude with implications for augmenting or innovating new MPP technologies. - "Enhancing Mobile Voice Assistants with WorldGaze"
-
Sven Mayer, Gierad Laput, Chris Harrison
Abstract:
Contemporary voice assistants require that objects of interest be specified in spoken commands. Of course, users are often looking directly at the object or place of interest – fine-grained, contextual information that is currently unused. We present WorldGaze, a software-only method for smartphones that provides the real-world gaze location of a user that voice agents can utilize for rapid, natural, and precise interactions. We achieve this by simultaneously opening the front and rear cameras of a smartphone. The front-facing camera is used to track the head in 3D, including estimating its direction vector. As the geometry of the front and back cameras are fixed and known, we can raycast the head vector into the 3D world scene as captured by the rear-facing camera. This allows the user to intuitively define an object or region of interest using their head gaze. We started our investigations with a qualitative exploration of competing methods, before developing a functional, real-time implementation. We conclude with an evaluation that shows WorldGaze can be quick and accurate, opening new multimodal gaze+voice interactions for mobile voice agents.Video:
- "Eyes on the Road: Detecting Phone Usage by Drivers Using On-Device Cameras"
-
Abstract:
Using a phone while driving is distracting and dangerous. It increases the accident chances by 400%. Several techniques have been proposed in the past to detect driver distraction due to phone usage. However, such techniques usually require instrumenting the user or the car with custom hardware. While detecting phone usage in the car can be done by using the phone's GPS, it is harder to identify whether the phone is used by the driver or one of the passengers. In this paper, we present a lightweight, software-only solution that uses the phone's camera to observe the car's interior geometry to distinguish phone position and orientation. We then use this information to distinguish between driver and passenger phone use. We collected data in 16 different cars with 33 different users and achieved an overall accuracy of 94% when the phone is held in hand and 92.2% when the phone is docked (≤1 sec. delay). With just a software upgrade, this work can enable smartphones to proactively adapt to the user's context in the car and and substantially reduce distracted driving incidents.Video:
- "Factors Influencing Perceived Fairness in Algorithmic Decision-Making: Algorithm Outcomes, Development Procedures, and Individual Differences"
-
Ruotong Wang, F. Maxwell Harper, Haiyi Zhu
Abstract:
Algorithmic decision-making systems are increasingly used throughout the public and private sectors to make important decisions or assist humans in making these decisions with real social consequences. While there has been substantial research in recent years to build fair decision-making algorithms, there has been less research seeking to understand the factors that affect people's perceptions of fairness in these systems, which we argue is also important for their broader acceptance. In this research, we conduct an online experiment to better understand perceptions of fairness, focusing on three sets of factors: algorithm outcomes, algorithm development and deployment procedures, and individual differences. We find that people rate the algorithm as more fair when the algorithm predicts in their favor, even surpassing the negative effects of describing algorithms that are very biased against particular demographic groups. We find that this effect is moderated by several variables, including participants' education level, gender, and several aspects of the development procedure. Our findings suggest that systems that evaluate algorithmic fairness through users' feedback must consider the possibility of "outcome favorability" bias. - "FitByte: Automatic Diet Monitoring in Unconstrained Situations Using Multimodal Sensing on Eyeglasses"
-
Abdelkareem Bedri, Diana Li, Rushil Khurana, Kunal Bhuwalka, Mayank Goel
Abstract:
In an attempt to help users reach their health goals and practitioners understand the relationship between diet and disease, researchers have proposed many wearable systems to automatically monitor food consumption. When a person consumes food, he/she brings the food close to their mouth, take a sip or bite and chew, and then swallow.Most diet monitoring approaches focus on one of these aspects of food intake, but this narrow reliance requires high precision and often fails in noisy and unconstrained situations common in a person's daily life. In this paper, we introduce FitByte, a multi-modal sensing approach on a pair of eyeglasses that tracks all phases of food intake. FitByte contains a set of inertial and optical sensors that allow it to reliably detect food intake events in noisy environments. It also has an on-board camera that opportunistically captures visuals of the food as the user consumes it. We evaluated the system in two studies with decreasing environmental constraints with 23 participants. On average, FitByte achieved 89% F1-score in detecting eating and drinking episodes. - "I'm All Eyes and Ears: Exploring Effective Locators for Privacy Awareness in IoT Scenarios"
-
Yunpeng Song, Yun Huang, Zhongmin Cai, Jason I. Hong
Abstract:
With the proliferation of IoT devices, there are growing concerns about being sensed or monitored by these devices unawares, especially in places perceived as private. We explore the design space of IoT locators to help people physically find nearby IoT devices. We first conducted a survey to understand people's willingness, current practices, and challenges in finding IoT devices. Our survey findings motivated us to design and implement low-cost locators (visual, auditory, and contextualized pictures) to help people find nearby devices. Through an iterative design process and two rounds of experiments, we found that these locators greatly reduced people's search time over a baseline of no locators. Many participants found the visual and auditory locators enjoyable. Some participants also appropriated the use of our system for other purposes, e.g., to learn about new IoT devices, instead of for privacy awareness. - "Improving Humans' Ability to Interpret Deictic Gestures in Virtual Reality"
-
Sven Mayer, Jens Reinhardt, Robin Schweigert, Brighten Jelke, Valentin Schwind, Katrin Wold, Niels Henze
Abstract:
Collaborative Virtual Environments (CVEs) offer unique opportunities for human communication. Humans can interact with each other over a distance in any environment and visual embodiment they want. Although deictic gestures are especially important as they can guide other humans' attention, humans make systematic errors when using and interpreting them. Recent work suggests that the interpretation of vertical deictic gestures can be significantly improved by warping the pointing arm. In this paper, we extend previous work by showing that models enable to also improve the interpretation of deictic gestures at targets all around the user. Through a study with 28 participants in a CVE, we analyzed the errors users make when interpreting deictic gestures. We derived a model that rotates the arm of a pointing user's avatar to improve the observing users' accuracy. A second study with 24 participants shows that we can improve observers' accuracy by 22.9%. As our approach is not noticeable for users, it improves their accuracy without requiring them to learn a new interaction technique or distracting from the experience.Video:
- "Informing the Design of a Personalized Privacy Assistant for the Internet of Things"
-
Jessica Colnago, Yuanyuan Feng, Tharangini Palanivel, Sarah Pearman, Megan Ung, Alessandro Acquisti, Lorrie Cranor, Norman Sadeh
Abstract:
Internet of Things (IoT) devices create new ways through which personal data is collected and processed by service providers. Frequently, end users have little awareness of, and even less control over, these devices' data collection. IoT Personalized Privacy Assistants (PPAs) can help overcome this issue by helping users discover and, when available, control the data collection practices of nearby IoT resources. We use semi-structured interviews with 17 participants to explore user perceptions of three increasingly more autonomous potential implementations of PPAs, identifying benefits and issues associated with each implementation. We find that participants weigh the desire for control against the fear of cognitive overload. We recommend solutions that address users' differing automation preferences and reduce notification overload. We discuss open issues related to opting out from public data collections, automated consent, the phenomenon of user resignation, and designing PPAs with at-risk communities in mind. - "Infrastructural Speculations: Tactics for Designing and Interrogating Lifeworlds"
-
Richmond Y. Wong, Vera Khovanskaya, Sarah E. Fox, Nick Merrill, Phoebe Sengers
Abstract:
This paper introduces "infrastructural speculations," an orientation toward speculative design that considers the complex and long-lived relationships of technologies with broader systems, beyond moments of immediate invention and design. As modes of speculation are increasingly used to interrogate questions of broad societal concern, it is pertinent to develop an orientation that foregrounds the "lifeworld" of artifacts—the social, perceptual, and political environment in which they exist. While speculative designs often imply a lifeworld, infrastructural speculations place lifeworlds at the center of design concern, calling attention to the cultural, regulatory, environmental, and repair conditions that enable and surround particular future visions. By articulating connections and affinities between speculative design and infrastructure studies research, we contribute a set of design tactics for producing infrastructural speculations. These tactics help design researchers interrogate the complex and ongoing entanglements among technologies, institutions, practices, and systems of power when gauging the stakes of alternate lifeworlds. - "It Takes a Village: Integrating an Adaptive Chatbot into an Online Gaming Community"
-
Joseph Seering, Michal Luria, Connie Ye, Geoff Kaufman, Jessica Hammer
Abstract:
While the majority of research in chatbot design has focused on creating chatbots that engage with users one-on-one, less work has focused on the design of conversational agents for online communities. In this paper we present results from a three week test of a social chatbot in an established online community. During this study, the chatbot "grew up" from "birth" through its teenage years, engaging with community members and "learning" vocabulary from their conversations. We discuss the design of this chatbot, how users' interactions with it evolved over the course of the study, and how it impacted the community as a whole. We discuss how we addressed challenges in developing a chatbot whose vocabulary could be shaped by users, and conclude with implications for the role of machine learning in social interactions in online communities and potential future directions for design of community-based chatbots. - "It's a scavenger hunt": Usability of Websites' Opt-Out and Data Deletion Choices
-
Hana Habib, Sarah Pearman, Jiamin Wang, Yixin Zou, Alessandro Acquisti, Lorrie Cranor, Norman Sadeh, Florian Schaub
Abstract:
"We conducted an in-lab user study with 24 participants to explore the usefulness and usability of privacy choices offered by websites. Participants were asked to find and use choices related to email marketing, targeted advertising, or data deletion on a set of nine websites that differed in terms of where and how these choices were presented. They struggled with several aspects of the interaction, such as selecting the correct page from a site's navigation menu and understanding what information to include in written opt-out requests. Participants found mechanisms located in account settings pages easier to use than options contained in privacy policies, but many still consulted help pages or sent email to request assistance. Our findings indicate that, despite their prevalence, privacy choices like those examined in this study are difficult for consumers to exercise in practice. We provide design and policy recommendations for making these website opt-out and deletion choices more useful and usable for consumers." - "LIFT: Integrating Stakeholder Voices into Algorithmic Team Formation"
-
Emily M. Hastings, Albatool Alamri, Andrew Kuznetsov, Christine Pisarczyk, Karrie Karahalios, Darko Marinov, Brian P. Bailey
Abstract:
Team formation tools assume instructors should configure the criteria for creating teams, precluding students from participating in a process affecting their learning experience. We propose LIFT, a novel learner-centered workflow where students propose, vote for, and weigh the criteria used as inputs to the team formation algorithm. We conducted an experiment (N=289) comparing LIFT to the usual instructor-led process, and interviewed participants to evaluate their perceptions of LIFT and its outcomes. Learners proposed novel criteria not included in existing algorithmic tools, such as organizational style. They avoided criteria like gender and GPA that instructors frequently select, and preferred those promoting efficient collaboration. LIFT led to team outcomes comparable to those achieved by the instructor-led approach, and teams valued having control of the team formation process. We provide instructors and designers with a workflow and evidence supporting giving learners control of the algorithmic process used for grouping them into teams. - "Pedestrian Detection with Wearable Cameras for the Blind: A Two-way Perspective"
-
Kyungjun Lee, Daisuke Sato, Saki Asakawa, Hernisa Kacorri, Chieko Asakawa
Abstract:
Blind people have limited access to information about their surroundings, which is important for ensuring one's safety, managing social interactions, and identifying approaching pedestrians. With advances in computer vision, wearable cameras can provide equitable access to such information. However, the always-on nature of these assistive technologies poses privacy concerns for parties that may get recorded. We explore this tension from both perspectives, those of sighted passersby and blind users, taking into account camera visibility, in-person versus remote experience, and extracted visual information. We conduct two studies: an online survey with MTurkers (N=206) and an in-person experience study between pairs of blind (N=10) and sighted (N=40) participants, where blind participants wear a working prototype for pedestrian detection and pass by sighted participants. Our results suggest that both of the perspectives of users and bystanders and the several factors mentioned above need to be carefully considered to mitigate potential social tensions. - "Proximate Social Factors in First-Time Contribution to Online Communities"
-
Joseph Seering, Jessica Hammer, Geoff Kaufman, Diyi Yang
Abstract:
In the course of every member's integration into an online community, a decision must be made to participate for the first time. The challenges of effective recruitment, management, and retention of new users have been extensively explored in social computing research. However, little work has looked at in-the-moment factors that lead users to decide to participate instead of "lurk", conditions which can be shaped to draw new users in at crucial moments. In this work we analyze 183 million messages scraped from chatrooms on the livestreaming platform Twitch in order to understand differences between first-time participants' and regulars' behaviors and to identify conditions that encourage first-time participation. We find that presence of diverse types of users increases likelihood of new participation, with effects depending on the size of the community. We also find that information-seeking behaviors in first-time participation are negatively associated with retention in the short and medium term. - "Race, Gender and Beauty: The Effect of Information Provision on Online Hiring Biases"
-
Weiwen Leung, Zheng Zhang, Daviti Jibuti, Jinhao Zhao, Maximilian Klein, Casey Pierce, Lionel Robert, Haiyi Zhu
Abstract:
We conduct a study of hiring bias on a simulation platform where we ask Amazon MTurk participants to make hiring decisions for a mathematically intensive task. Our findings suggest hiring biases against Black workers and less attractive workers, and preferences towards Asian workers, female workers and more attractive workers. We also show that certain UI designs, including provision of candidates' information at the individual level and reducing the number of choices, can significantly reduce discrimination. However, provision of candidate's information at the subgroup level can increase discrimination. The results have practical implications for designing better online freelance marketplaces. - "Social Boundaries for Personal Agents in the Interpersonal Space of the Home"
-
Michal Luria, Rebecca Zheng, Bennett Huffman, Shuangni Huang, John Zimmerman, Jodi Forlizzi
Abstract:
The presence of voice activated personal assistants (VAPAs) in people's homes rises each year [31]. Industry efforts are invested in making interactions with VAPAs more personal by leveraging information from messages and calendars, and by accessing user accounts for 3rd party services. However, the use of personal data becomes more complicated in interpersonal spaces, such as people's homes. Should a shared agent access the information of many users? If it does, how should it navigate issues of privacy and control? Designers currently lack guidelines to help them design appropriate agent behaviors. We used Speed Dating to explore inchoate social mores around agent actions within a home, including issues of proactivity, interpersonal conflict, and agent prevarication. Findings offer new insights on how more socially sophisticated agents might sense, make judgements about, and navigate social roles and individuals. We discuss how our findings might impact future research and future agent behaviors. - "The TA Framework: Designing Real-time Teaching Augmentation for K-12 Classrooms"
-
Pengcheng An, Kenneth Holstein, Bernice d'Anjou, Berry Eggen, Saski Bakker
Abstract:
Recently, the HCI community has seen increased interest in the design of teaching augmentation (TA): tools that extend and complement teachers' pedagogical abilities during ongoing classroom activities. Examples of TA systems are emerging across multiple disciplines, taking various forms: e.g., ambient displays, wearables, or learning analytics dashboards. However, these diverse examples have not been analyzed together to derive more fundamental insights into the design of teaching augmentation. Addressing this opportunity, we broadly synthesize existing cases to propose the TA framework. Our framework specifies a rich design space in five dimensions, to support the design and analysis of teaching augmentation. We contextualize the framework using existing designs cases, to surface underlying design trade-offs: for example, balancing actionability of presented information with teachers' needs for professional autonomy, or balancing unobtrusiveness with informativeness in the design of TA systems. Applying the TA framework, we identify opportunities for future research and design. - "The Upcycled Home: Removing Barriers to Lightweight Modification of the Home's Everyday Objects"
-
Kristin Williams, Rajitha Pulivarthy, Scott E. Hudson, Jessica Hammer
Abstract:
The Internet-of-things (IoT) embeds computing in everyday objects, but has largely focused on new devices while ignoring the home's many existing possessions. We present a field study with 10 American families to understand how these possessions could be included in the smart home through upcycling. We describe three patterns for how families collaborate around home responsibilities; we explore families' mental models of home that may be in tension with existing IoT systems; and we identify ways that families can more easily imagine a smart home that includes their existing possessions. These insights can help us design an upcycled approach to IoT that supports users in reconfiguring objects (and social roles as mediated by objects) in a way that is sensitive to what will be displaced, discarded, or made obsolete. Our findings inform the design of future lightweight systems for the upcycled home. - "Towards Value-Adaptive Instruction: A Data-Driven Method for Addressing Bias in Argument Evaluation Tasks"
-
Nicholas Diana, John Stamper, Ken R. Koedinger
Abstract:
As the media landscape is increasingly populated by less than reputable sources of information, educators have turned to argument evaluation training as a potential solution. Unfortunately, the bias literature suggests that our ability to objectively evaluate an argument is, to a large extent, determined by the relationship between our own beliefs and the beliefs latent in the argument we are evaluating. If the argument supports our worldview, we are much more likely to overlook logical errors. Teachers recognize this need to adapt argument evaluation instruction to the specific beliefs of students. For instance, a teacher might intentionally assign a student an argument that the student disagrees with. Unfortunately, this kind of value-adaptive instruction is infrequent due to its unscalability. We propose a novel method for data-driven value-adaptive instruction in instructional technologies. This method can be used to combat bias in real-world contexts and support human reasoning during media consumption. - "Understanding and Visualizing Data Iteration in Machine Learning"
-
Fred Hohman, Kanit Wongsuphasawat, Mary Beth Kery, Kayur Patel
Abstract:
Successful machine learning (ML) applications require iterations on both modeling and the underlying data. While prior visualization tools for ML primarily focus on modeling, our interviews with 23 ML practitioners reveal that they improve model performance frequently by iterating on their data (e.g., collecting new data, adding labels) rather than their models. We also identify common types of data iterations and associated analysis tasks and challenges. To help attribute data iterations to model performance, we design a collection of interactive visualizations and integrate them into a prototype, Chameleon, that lets users compare data features, training/testing splits, and performance across data versions. We present two case studies where developers apply \system to their own evolving datasets on production ML projects. Our interface helps them verify data collection efforts, find failure cases stretching across data versions, capture data processing changes that impacted performance, and identify opportunities for future data iterations. - "Understanding Client Support Strategies to Improve Clinical Outcomes in an Online Mental Health Intervention"
-
Prerna Chikersal, Danielle Belgrave, Gavin Doherty, Angel Enrique, Jorge E. Palacios, Derek Richards, Anja Thieme
Abstract:
Online mental health interventions are increasingly important in providing access to, and supporting the effectiveness of, mental health treatment. While these technologies are effective, user attrition and early disengagement are key challenges. Evidence suggests that integrating a human supporter into such services mitigates these challenges, however, it remains under-studied how supporter involvement benefits client outcomes, and how to maximize such effects. We present our analysis of 234,735 supporter messages to discover how different support strategies correlate with clinical outcomes. We describe our machine learning methods for: (i) clustering supporters based on client outcomes; (ii) extracting and analyzing linguistic features from supporter messages; and (iii) identifying context-specific patterns of support. Our findings indicate that concrete, positive and supportive feedback from supporters that reference social behaviors are strongly associated with better outcomes; and show how their importance varies dependent on different client situations. We discuss design implications for personalized support and supporter interfaces. - "Understanding User Perception of Automated News Generation System"
-
Changhoon Oh, Jinhan Choi, Sungwoo Lee, SoHyun Park, Daeryong Kim, Jungwoo Song, Dongwhan Kim, Joonhwan Lee, Bongwon Suh
Abstract:
Automated journalism refers to the generation of news articles using computer programs. Although it is widely used in practice, its user experience and interface design remain largely unexplored. To understand the user perception of an automated news system, we designed NewsRobot, a research prototype that automatically generated news on major events of the PyeongChang 2018 Winter Olympic Games in real-time. It produces six types of news by combining two kinds of content (general/individualized) and three styles (text, text+image, text+image+sound). A total of 30 users participated in using NewsRobot, completing surveys and interviews on their experience. Our findings are as follows: (1) Users preferred individualized news yet considered it less credible, (2) more presentation elements were appreciated but only if their quality was assured, and (3) NewsRobot was considered factual and accurate yet shallow in depth. Based on our findings, we discuss implications for designing automated journalism user interfaces. - "A Case for Humans-in-the-Loop: Decisions in the Presence of Erroneous Algorithmic Scores"
-
Maria De-Arteaga, Riccardo Fogliato, Alexandra Chouldechova
Abstract:
The increased use of algorithmic predictions in sensitive domains has been accompanied by both enthusiasm and concern. To understand the opportunities and risks of these technologies, it is key to study how experts alter their decisions when using such tools. In this paper, we study the adoption of an algorithmic tool used to assist child maltreatment hotline screening decisions. We focus on the question: Are humans capable of identifying cases in which the machine is wrong, and of overriding those recommendations? We first show that humans do alter their behavior when the tool is deployed. Then, we show that humans are less likely to adhere to the machine's recommendation when the score displayed is an incorrect estimate of risk, even when overriding the recommendation requires supervisory approval. These results highlight the risks of full automation and the importance of designing decision pipelines that provide humans with autonomy.