HCII Seminar Series - Jorge Ortiz
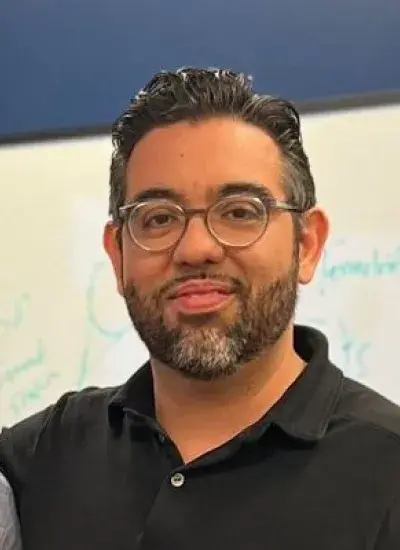
Speaker
Jorge Ortiz
Assistant Professor, Electrical and Computer Engineering, Rutgers University
When
-
Where
Newell-Simon Hall 1305
Video
Panopto
Description
"Advancing Human-Machine Interactions: Multimodal Learning in Densely Sensed Spaces"
The proliferation of ubiquitous computing and instrumented environments has catalyzed multimodal learning as a pivotal paradigm for enhancing real-world applications and interactions. From autonomous vehicles traversing intricate urban landscapes to assistive robots in healthcare settings, seamless integration of heterogeneous sensor data is imperative. Multimodal learning harnesses this wealth of sensory information to construct nuanced computational models that surpass unimodal counterparts in accuracy, context-awareness, and user engagement.
This talk focuses on multimodal learning in heterogeneous, densely sensed environments, examining its applications in interactive systems like vehicles and robots, enhancing inference and model interpretability through our GeXSe framework, and extending its utility to outdoor interactions via our work with the Center for Smart Streetscapes (CS3). We start by showcasing how sensor fusion techniques create robust contextual embeddings to improve the timing of interactions in vehicular systems significantly. With an F1-score of 0.874, our approach predicts optimal moments for in-car speech interaction. Additionally, our Social Nibbling Network model (SoNNet) surpasses fixed-interval strategies in a robot-feeding scenario, backed by a dining study that establishes user preference for our model. Next, we introduce GeXSe (Generative Explanatory Sensor System), a framework designed to provide interpretability in multimodal learning models. Through parallel multi-branch MLP and generative modeling techniques, GeXSe outperforms not only baseline metrics but also bolsters model interpretability by offering visual explanations derived from heterogeneous sensor data. Finally, we describe applications of our research in densely-sensed urban settings, notably through the NSF-funded Center for Smart Streetscapes (CS3). Here, we explore the integration of hyperlocal data and multimodal inference in outdoor interactive applications. The talk aims to highlight the critical challenges and immense potential of applying fine-grained spatiotemporal data in crafting intelligent, context-aware interactive systems.
Speaker's Bio
Jorge Ortiz is an assistant professor of Electrical and Computer Engineering at Rutgers University. His expertise lies in sensing systems, interaction, and multimodal learning within heterogeneous, densely sensed environments. Dr. Ortiz serves as a Principal Investigator on the recently awarded $26 million NSF Engineering Research Center, the Center for Smart Streetscapes (CS3), which focuses on advancing multimodal inference in outdoor environments. Prior to his academic role at Rutgers, he was a research staff member at IBM Research, where he attained over a dozen patents in machine learning-based IoT systems. Dr. Ortiz earned his M.S. and Ph.D. in Computer Science from the University of California, Berkeley, in 2010 and 2013, respectively, and received his B.S. in Computer Science and Engineering from the Massachusetts Institute of Technology in 2003.
Speaker's Website
http://jorgeortizphd.info/