Related People
Mayank Goel, Rushil Khurana
SMASH Lab Uses Wearables To Train New Privacy-Preserving Sensors
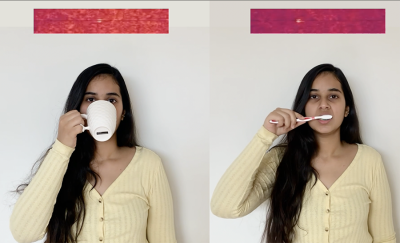

Data about home sales likely won't help someone looking for a car, just like information about basketball won't help someone playing baseball.
But that's not true inside the Smart Sensing for Humans (SMASH) Lab at Carnegie Mellon University, where researchers use data collected from one type of sensor to train another. Their work, called IMU2Doppler, has shown that data collected from inertial measurement unit (IMU) sensors in smartwatches can quickly train a millimeter wave doppler to recognize human movements and behaviors.
Doppler sensors use millimeter waves and the doppler effect to determine the velocity and direction of a moving object. The ambient sensor can be installed in a smart house, where it can recognize and track daily activities such as eating, drinking, brushing teeth and folding clothes. It is a privacy-preserving alternative to popular smart devices with speakers and cameras that have raised concerns about privacy.
While large, labeled data sets exist for training sensors that depend on IMU or video, they do not exist for doppler sensors. Collecting and labeling the data takes time and resources — expenditures researchers could avoid through the work of the SMASH Lab team.
"Wearables like Fitbit and Apple Watch have been commonplace for so many years now. People have already put so much time into labeling IMU data sets for everyday activities, and we can just piggyback on that to label new sensors such as a doppler." said Rushil Khurana, a member of SMASH Lab and Ph.D. student in human-computer interaction.
The team used a neural network trained on existing IMU and video data to facilitate training a doppler sensor model, and found that it was 75% accurate right out of the gate with only a few seconds of its own training data. This effort builds on previous work in video and audio.
Besides tracking daily activities in a house, researchers in the SMASH Lab eventually want to track and monitor the movements of people in their own homes to help doctors, counselors, clinicians and other medical professionals spot illnesses or the signs of potential problems. Doppler sensors installed in people's homes could make this possible.
"We want to link a person's activities and behaviors in their homes to their physical and mental health. We could help diagnose depression, sleep fatigue and other conditions." said Mayank Goel, who heads the SMASH Lab and is an assistant professor in the Institute for Software Research and the Human-Computer Interaction Institute.
Aaron Aupperlee | 412-268-9068 | aaupperlee@cmu.edu
Research Areas