Wikibench
Community-Driven Data Curation for AI Evaluation on Wikipedia
2024
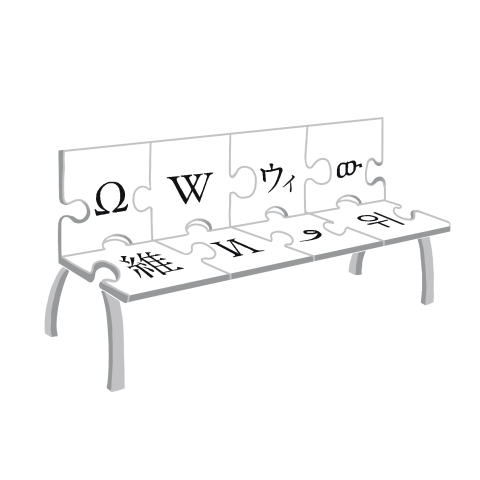
Wikibench is a system that enables communities to collaboratively curate AI datasets, while navigating ambiguities and disagreements through discussion.
AI tools are increasingly deployed in community contexts. However, datasets used to evaluate AI are typically created by developers and annotators outside a given community, which can yield misleading conclusions about AI performance. How might we empower communities to drive the intentional design and curation of evaluation datasets for AI that impacts them? We investigate this question on Wikipedia, an online community with multiple AI-based content moderation tools deployed. We introduce Wikibench, a system that enables communities to collaboratively curate AI evaluation datasets, while navigating ambiguities and differences in perspective through discussion. A field study on Wikipedia shows that datasets curated using Wikibench can effectively capture community consensus, disagreement, and uncertainty. Furthermore, study participants used Wikibench to shape the overall data curation process, including refining label definitions, determining data inclusion criteria, and authoring data statements. Based on our findings, we propose future directions for systems that support community-driven data curation.
Learn more on the arXiv page: Wikibench
Researchers
Tzu-Sheng Kuo, Sherry Tongshuang Wu, Ken Holstein, Haiyi Zhu
Research Areas